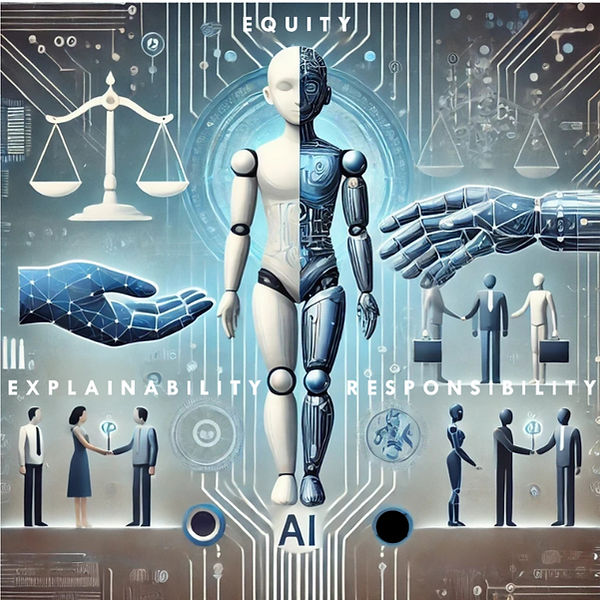
Navigating Ethical Dilemmas in AI-Driven Risk Management: Explainability, Equity, and Responsibility
by Dr. Anton Gates
August 29, 2024
Introduction to Navigating Ethical Dilemmas
As AI systems become integral to project risk management, they introduce new ethical challenges that must be addressed to maintain trust and integrity in decision-making (Guan et al., 2022). Ethical dilemmas in AI-driven risk management arise when the benefits of automation and predictive capabilities conflict with potential risks, such as biased outcomes, lack of transparency, or accountability issues. Addressing these dilemmas is not merely a technical task but a moral imperative that ensures that AI systems align with and uphold the societal values of fairness, equality, and justice, contributing positively to project outcomes (Jobin et al., 2019).
However, this raises a critical question: Are we merely creating the illusion of ethical AI by focusing on explainability and fairness? Despite significant efforts to enhance transparency and equity, AI systems may still inherently reflect the biases and limitations of their creators (Abbu et al., 2022). If AI's ethicality is only as deep as the biases embedded in its training data, then the very foundation of AI ethics warrants closer examination.
Explainability
Why Explainability Matters
Explainability refers to the clarity with which human stakeholders can understand an AI system’s decision-making process. In the context of risk management, where decisions can significantly impact project outcomes, the need for transparent AI models is paramount. Without explainability, stakeholders may struggle to trust AI-enabled systems (Maclure, 2021), particularly when decisions appear counterintuitive or when AI identifies risks that were previously unrecognized. Building this trust is a critical factor in implementing AI-enabled systems.
Techniques for Enhancing Transparency
Several techniques can make AI models more transparent:
-
Feature Importance Analysis: This method helps identify which variables most influence AI's decisions, offering insights into the model's functioning (Gebreyesus et al., 2024).
-
Model-Agnostic Methods: Techniques like LIME (Local Interpretable Model-Agnostic Explanations) and SHAP (SHapley Additive exPlanations) can be applied to any model, providing understandable explanations without altering the underlying algorithm (Lee et al., 2023).
-
Visualization Tools: Graphical representations of decision processes, such as decision trees or heat maps, can also aid in demystifying complex AI models (Villegas-Ch et al., 2023).
Trade-offs Between Transparency and Complexity
A significant challenge in AI is balancing the need for transparency with the inherent complexity of advanced models (Zerilli et al., 2018). Often, the most powerful AI models, such as deep neural networks, are also the least transparent. Simplifying these models to enhance explainability might reduce their predictive accuracy. It is essential to consider whether the pursuit of transparency could undermine the power that makes AI transformative. In prioritizing explainability, are we sacrificing effectiveness, potentially exposing projects to more significant risks? This trade-off challenges us to weigh the value of a model's understandability against its capacity to deliver superior outcomes, even if that means accepting the "black box" nature of AI.
Equity
Ensuring Fairness in AI Models
Equity in AI-driven risk management means ensuring that AI systems do not perpetuate or exacerbate existing biases. Fairness is especially critical when AI decisions affect diverse groups, as biased models can lead to unfair treatment, such as unequal risk assessments across different demographics. Achieving equity requires a concerted effort to identify and mitigate biases in AI models.
Cultural Nuances and Inherent Biases
However, a more profound challenge lies in the fact that cultures themselves have inherent biases. These biases are often deeply rooted in historical, social, and economic contexts and can be difficult to fully understand, especially for those outside the culture (Binns, 2018). Is it reasonable to expect that mindfulness of cultural nuances can elicit anything other than bias in the very act of mindfulness? This question highlights the paradox of trying to address cultural biases: the more we attempt to account for cultural differences, the more we might inadvertently reinforce the biases associated with those differences.
This dilemma is particularly problematic in AI-driven risk management, where the goal is to create fair and equitable systems across all cultural contexts. However, the very act of incorporating cultural nuances into AI models can lead to biased outcomes if those nuances are misinterpreted or oversimplified by the algorithms (Green & Viljoen, 2020).
Addressing the Dilemma
To effectively address this dilemma and produce true equity in AI-driven risk management, several strategies can be employed:
-
Incorporate Diverse Perspectives: AI development teams must include individuals from a wide range of cultural backgrounds who can provide insights into how cultural nuances might influence AI outcomes (Buolamwini & Gebru, 2018). This diversity can help prevent the oversimplification or misinterpretation of cultural factors.
-
Engage in Continuous Learning and Feedback: AI systems should be designed to learn continuously from their interactions with different cultural contexts. This means regularly updating the models based on real-world feedback and ensuring that AI evolves as it encounters new cultural information (Leslie, 2019).
-
Utilize Context-Sensitive Algorithms: AI models should be designed to adapt to specific cultural contexts instead of applying a one-size-fits-all approach (Barocas et al., 2023). Context-sensitive algorithms can help ensure that decisions are made with an understanding of the cultural nuances relevant to each situation.
-
Foster Transparency and Accountability: Transparency in how AI systems account for cultural differences is crucial. Organizations should be clear about the limitations of their AI models and the steps they are taking to mitigate cultural biases (Ananny & Crawford, 2016). Additionally, accountability mechanisms should be in place to address any unintended consequences arising from cultural misinterpretations.
By acknowledging the inherent challenges in accounting for cultural nuances and actively mitigating the biases that may arise from such efforts, organizations can move closer to achieving true equity in AI-driven risk management. This requires a commitment to continuous learning, diverse perspectives, and developing more sophisticated, context-aware algorithms.
The Role of Diverse Datasets
Diverse and representative datasets are the cornerstone of fair AI systems. Project leaders can reduce the likelihood of biased outcomes by training models on data that reflects the full spectrum of the populations they will impact (Gebru et al., 2021). It is also essential to continuously audit these datasets to ensure they remain representative as the project context evolves.
Addressing Systemic Biases
Systemic biases, often ingrained in historical data, can be inadvertently encoded into AI models. Addressing these biases requires technical solutions, such as fairness-aware algorithms and a commitment to ethical data practices (Hagendorff, 2022). Regular audits and bias detection tools are critical for identifying and correcting inequities in AI-driven decisions.
Responsibility
Ethical Responsibility of Organizations
Organizations deploying AI for risk management have a profound ethical responsibility to ensure their systems operate fairly, transparently, and safely. This responsibility extends beyond the technical aspects of AI to include the broader social and ethical implications of its use (Floridi & Cowls, 2022). Ethical responsibility involves establishing clear accountability frameworks for AI decisions and ensuring that these frameworks are aligned with the organization’s values.
Accountability Mechanisms
To manage AI responsibly, organizations must implement robust accountability mechanisms (Raji & Buolamwini, 2019). These mechanisms should clearly define who is responsible for AI system outputs, how issues will be addressed if they arise, and what governance structures will oversee the ethical use of AI. Tools like automated machine learning platforms, which include built-in ethical safeguards, can support these efforts.
As AI systems increasingly take on autonomous roles in risk management, there is a growing concern that we might be outsourcing too much responsibility to machines. The more we rely on AI for critical decisions, the less accountable human leaders may become. This shift raises a significant issue: Could this delegation of responsibility lead to a leadership crisis where the line between human and machine accountability blurs beyond recognition?
Prediction: The Future of Accountability in AI-Driven Risk Management
As AI continues to evolve and become more autonomous, traditional frameworks of accountability may be fundamentally challenged. Within the next decade, we may witness a shift where AI-driven decisions are accepted and legally recognized as independent entities in risk management. This could lead to a scenario where AI systems are granted a form of "operational autonomy," effectively blurring the lines between machine and human accountability.
However, this raises a profound and contentious question: Should AI systems be granted the same legal and ethical status as human decision-makers? If AI begins to operate with such autonomy, could we be paving the way for AI to assume roles traditionally reserved for humans, potentially even superseding human authority in critical decision-making processes? This scenario challenges the very essence of what it means to be accountable and who—or what—should ultimately hold that responsibility.
Moreover, as AI systems gain complexity, sophistication, and even a form of creativity, it is inevitable that the legality of algorithmic decision-making—particularly decisions that result in adverse outcomes—will be rigorously challenged. The question of ownership will also come to the forefront: Who owns the favorable outcomes, such as innovations or significant financial windfalls, resulting from autonomous AI decision-making? These challenges could lead to unprecedented legal disputes, reshaping our understanding of intellectual property, responsibility, and the ethical implications of AI-driven success.
Real-World Example: Ethical Dilemmas in AI Models
Case Study: Bias in AI-Driven Credit Risk Assessment
Consider the case of a financial institution that implemented an AI model to assess credit risk. The model was trained on historical data that included demographic information, inadvertently reflecting past discriminatory lending practices. As a result, the AI system began to perpetuate these biases, unfairly denying loans to specific demographic groups. The organization addressed this ethical dilemma by retraining the AI model using fairness-aware algorithms and more representative data. This case underscores the importance of focusing on explainability, equity, and responsibility to resolve ethical challenges in AI-driven risk management.
Conclusion
Navigating ethical dilemmas in AI-driven risk management is essential for ensuring that powerful AI-enabled tools contribute positively to project outcomes. By prioritizing explainability, equity, and responsibility, organizations can build AI systems that are effective and aligned with ethical standards. A proactive approach to ethics in AI will mitigate risks and enhance the overall trust and acceptance of AI-driven decisions among stakeholders. As AI continues to evolve, so must our commitment to navigating its ethical complexities with diligence and care.
References
Abbu, H., Mugge, P., & Gudergan, G. (2022). Ethical Considerations of Artificial Intelligence: Ensuring Fairness, Transparency, and
Explainability. 2022 IEEE 28th International Conference on Engineering, Technology and Innovation (ICE/ITMC) &Amp; 31st International Association for Management of Technology (IAMOT) Joint Conference. https://doi.org/10.1109/ice/itmc-iamot55089.2022.10033140
Ananny, M., & Crawford, K. (2016). Seeing without knowing: Limitations of the transparency ideal and its application to algorithmic
accountability. New Media & Society, 20(3), 973–989. https://doi.org/10.1177/1461444816676645
Barocas, S., Hardt, M., & Narayanan, A. (2023). Fairness and Machine Learning: Limitations and Opportunities. https://fairmlbook.org/
Binns, R. (2017, December 10). Fairness in Machine Learning: Lessons from Political Philosophy. arXiv.org.
http://arxiv.org/abs/1712.03586
Buolamwini, J., & Gebru, T. (2018, January 21). Gender Shades: Intersectional Accuracy Disparities in Commercial Gender
Classification. PMLR. https://proceedings.mlr.press/v81/buolamwini18a.html?mod=article_inline&ref=akusion-ci-shi-dai-bizinesumedeia
Floridi, L., & Cowls, J. (2022b). A Unified Framework of Five Principles for AI in Society. 535–545.
https://doi.org/10.1002/9781119815075.ch45
Gebreyesus, Y., Dalton, D., De Chiara, D., Chinnici, M., & Chinnici, A. (2024). AI for Automating Data Center Operations: Model
Explainability in the Data Centre Context Using Shapley Additive Explanations (SHAP). Electronics, 13(9), 1628. https://doi.org/10.3390/electronics13091628
Gebru, T., Morgenstern, J., Vecchione, B., Vaughan, J. W., Wallach, H., Daumé, H., & Crawford, K. (2021). Datasheets for datasets.
Communications of the ACM, 64(12), 86–92. https://doi.org/10.1145/3458723
Green, B., & Viljoen, S. (2020). Algorithmic realism. https://doi.org/10.1145/3351095.3372840
Guan, H., Dong, L., & Zhao, A. (2022). Ethical Risk Factors and Mechanisms in Artificial Intelligence Decision Making. Behavioral
Sciences (2076-328X), 12(9), 343. https://doi.org/10.3390/bs12090343
Hagendorff, T. (2020). The Ethics of AI Ethics: An Evaluation of Guidelines. Minds and Machines, 30(1), 99–120.
https://doi.org/10.1007/s11023-020-09517-8
Jobin, A., Ienca, M., & Vayena, E. (2019). The global landscape of AI ethics guidelines. Nature Machine Intelligence, 1(9),
389–399. https://doi.org/10.1038/s42256-019-0088-2
Lee, A. H. S., Shankararaman, V., & Ouh, E. L. (2023). Vision Paper: Advancing of AI Explainability for the Use of ChatGPT in
Government Agencies – Proposal of A 4-Step Framework. https://doi.org/10.1109/bigdata59044.2023.10386797
Leslie, D. (2019). Understanding artificial intelligence ethics and safety. arXiv (Cornell University).
https://doi.org/10.48550/arxiv.1906.05684
Maclure, J. (2021). AI, Explainability and Public Reason: The Argument from the Limitations of the Human Mind. Minds and Machines, 31(3), 421–438. https://doi.org/10.1007/s11023-021-09570-x
Raji, I. D., & Buolamwini, J. (2019). Actionable Auditing. https://doi.org/10.1145/3306618.3314244
Villegas-Ch, W., García-Ortiz, J., & Jaramillo-Alcazar, A. (2023). An Approach Based on Recurrent Neural Networks and Interactive
Visualization to Improve Explainability in AI Systems. Big Data and Cognitive Computing, 7(3), 136. https://doi.org/10.3390/bdcc7030136
Whittlestone, J., Nyrup, R., Alexandrova, A., & Cave, S. (2019). The Role and Limits of Principles in AI
Ethics. https://doi.org/10.1145/3306618.3314289
Zerilli, J., Knott, A., Maclaurin, J., & Gavaghan, C. (2018). Transparency in Algorithmic and Human Decision-Making: Is There a
Double Standard? Philosophy & Technology, 32(4), 661–683. https://doi.org/10.1007/s13347-018-0330-6
Dr. Anton Gates, DBA, MBA, PMP, MCPM, is an academic and researcher specializing in business strategy, digital transformation, and the evolving impacts of AI on organizations. With over 30 years of experience bridging industry practice and academic inquiry, Dr. Gates has authored numerous articles on the intersection of technology, education, and business. Explore more of his writings here: Articles and Publications