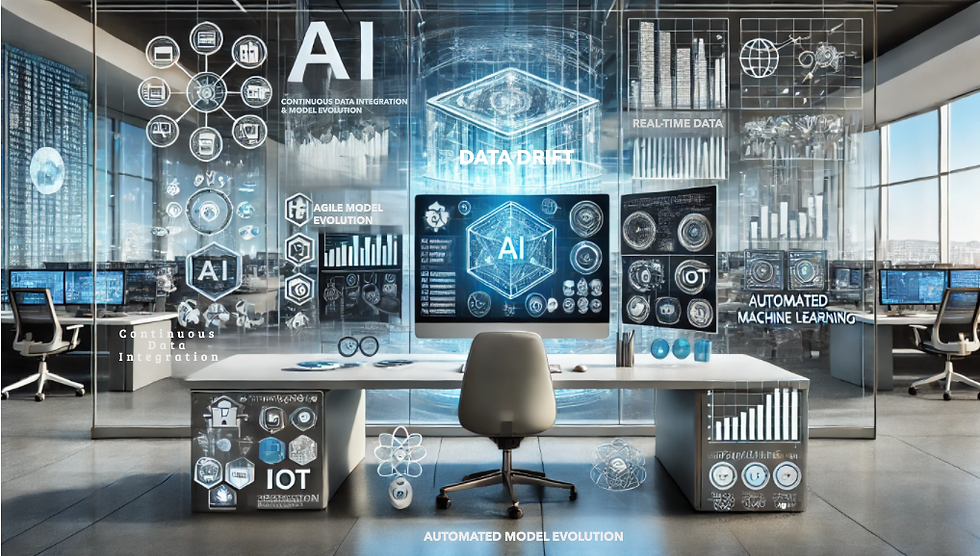
I are thrilled to announce the release of our latest article, "Risk Management in the AI Era: A Comprehensive Guide for Project Leaders - Model Evolution," a comprehensive guide for project leaders navigating the dynamic landscape of artificial intelligence.
What’s Inside?
In this insightful piece, Dr. Anton Gates delves into the critical role of continuous model evolution in AI-driven risk management. Here’s a sneak peek into the key themes covered:
1. Importance of Model Evolution
Discover why continuous improvement and adaptation of AI models are not just best practices but strategic necessities for maintaining accuracy and relevance in risk management.
2. Continuous Data Integration
Learn how integrating new data into existing AI models ensures they operate with the most current information, enhancing their predictive capabilities and reliability.
3. Regular Model Retraining
Understand the significance of regular retraining to adapt AI models to new data and evolving patterns, making them more efficient and accurate.
4. Agility in Model Evolution
Explore how combining AI with agile methodologies fosters a dynamic environment for rapid model iteration and improvement.
5. Monitoring and Evaluation
Gain insights into the essential techniques for continuous monitoring and evaluation to sustain model performance and effectiveness.
6. Leveraging Real-Time Data and Advanced Analytics
See how real-time data integration and advanced analytics are critical for maintaining AI model efficiency and accuracy.
7. Addressing Challenges in Model Evolution
Find out how to tackle common challenges such as data drift and computational costs to ensure robust AI model evolution.
8. Future Trends in Model Evolution
Stay ahead of the curve by learning about emerging technologies and methodologies like AutoML, XAI, and federated learning that are transforming model evolution.
9. Real-World Example: Data Center Project Risk Management
Read a detailed case study on how a technology company successfully implemented an AI-driven risk management system in a large-scale data center project.
Key Takeaways
"In AI-driven risk management, failing to evolve your models is akin to navigating with outdated maps—dangerous and irresponsible. Model evolution isn't just a best practice; it's a strategic necessity that defines the difference between success and failure." — Dr. Anton Gates
"Relying on static models in a dynamic world is a recipe for disaster. Continuous model evolution must be at the heart of any risk management strategy to ensure resilience and adaptability in the face of ever-changing risks." — Dr. Anton Gates
Why Read This Article?
This article is a must-read for project leaders, AI practitioners, and anyone involved in risk management. It provides practical insights, backed by real-world examples and academic references, to help you understand and implement effective AI-driven risk management strategies.
Read the Full Article
Dive into the full article to explore these insights and more: Read the Full Article Here
Share the Knowledge
If you find this article valuable, please share it with a friend or colleague who might benefit from these insights.
Stay tuned for more expert insights and practical guides from Dr. Anton Gates and our team at Executive Insight Solutions.
Comments